Winning teams see things others don't and do things others can't.
In finance, "alpha" is an investment’s outperformance over a market benchmark. It's what separates market-beating investors from the rest of the pack. Every GTM team is constantly seeking alpha—even if you don’t call it that yet. Each time you refine your targeting or messaging to beat your competitors, you're chasing alpha.
And just like investors, winning GTM teams use data others don't have—in plays others can’t run—to find an edge.
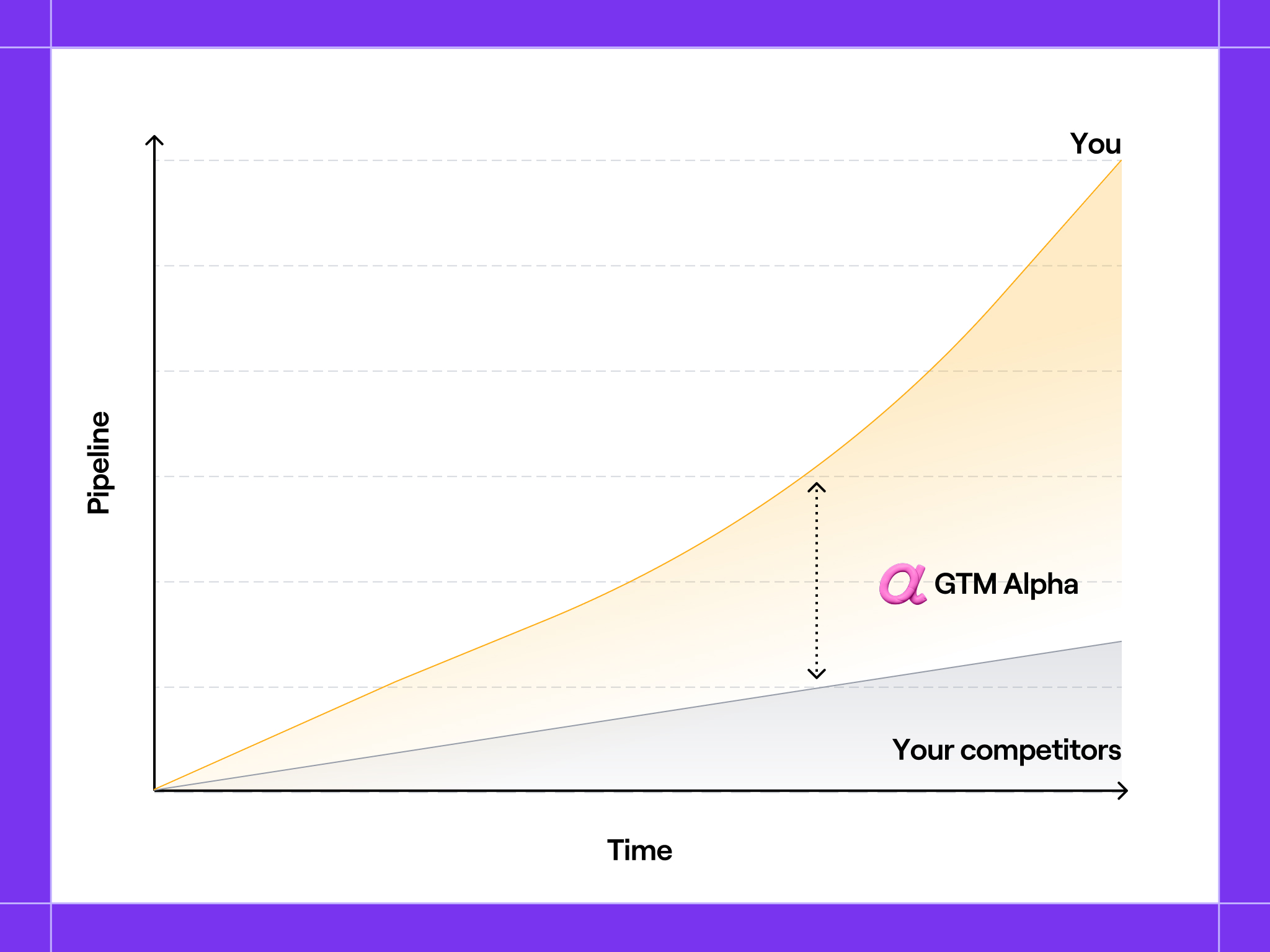
GTM alpha starts with better data than your competitors. Accurate, comprehensive, and timely data is table stakes, though most companies don’t even get this far. (If you can’t even trust the email addresses or firmographics in your CRM, fix that first.)
Unique data, however, is your competitive edge. While competitors blast messages to "all restaurants in Europe," you should be pinpointing Berlin cafes with $30-50 entrees that just joined Doordash. Instead of targeting generic SaaS companies, you should be finding those with usage-based pricing, free trials, and newly posted customer success jobs. Unique data gives you a precision edge.
Study your best customers to know what unique data points to look for. AI agents can find any data point at scale by reading websites or documents to categorize or summarize information. They can, for example, infer whether a company has specific certifications or exceeds a certain growth rate.
Armed with unique data, winning teams can run unique plays. Plays based on surface-level personalizations—like mentioning someone's college or referencing website visits—never work for long. Exceptional performers do it differently. A global design company, for example, has thought about using AI to find brand inconsistencies between prospects' websites and social media accounts and suggest solutions—an approach that performs twice as well as generic outreach.
Continuous experimentation is the key to maintaining alpha. No play lasts forever: the market and your customers are constantly changing. Top teams stay ahead by experimenting, learning, and launching better plays faster. By the time competitors reverse-engineer a strategy that worked for you last year, you should be on to your next one.

Part 1: Find your unique data advantage
When you and your competitors only target based on company size, industry, and job titles, you're all going to be selling to the same companies at the same time. Unique AI data—tailored to your company’s product and value proposition—will help you more precisely narrow in on accounts that have a burning need for your product. These data points are difficult to find, which means BDRs often spend their time manually researching them. But AI agents can systematically collect these qualitative & unstructured data points. They're the hidden signals that your competitors can't see because they don't know to look for them.
To decide what unique data points you need, the first step is to deeply understand your customers. Interview your sales team about what they manually research before calls and why those signals matter. Listen to where prospects are having conversations and what language they use. Map their digital footprint: what they read, where they gather, what tools they mention, and what problems they discuss. If you had 100 interns, what would you have them do? If you could reach out to a company at any specific time, when would it be? Most companies we’ve met, including market leaders, do not put nearly enough scrutiny into this.
The next step is turning these indicators of customer fit into unique data points you can get with AI. Ideally, this combines public data, 1st party data, as well as custom signals. For example, when a prospect told us they land the most deals right before businesses enter promotion cycles, we suggested they use AI to find employee promotion date clusters and map these to different firms.
Here are examples of how companies are using Clay’s AI agent to discover unique data points their competitors can't see:
- Certemy counts OSHA violations to find companies with compliance problems
- Supermetrics distinguishes between brands and agencies to route leads to the right sales teams
- Rutter uses AI to identify high-value executives who need financial products the moment relevant conference attendee lists become public
- Intercom determines the breadth of a company’s support documentation
- Cake.ai identifies AI engineering teams that have 2-5 developers (their sweet spot)
Other customers try novel ideas like scanning satellite images to analyze building locations for indicators of occupancy, tracking where prospects are expanding office locations, etc.
Unique data is most powerful when paired with precise timing. Markets change daily, and signals often have short windows of relevance. Companies that quickly capture and act on time-sensitive data consistently outperform those relying on monthly or quarterly refreshes.
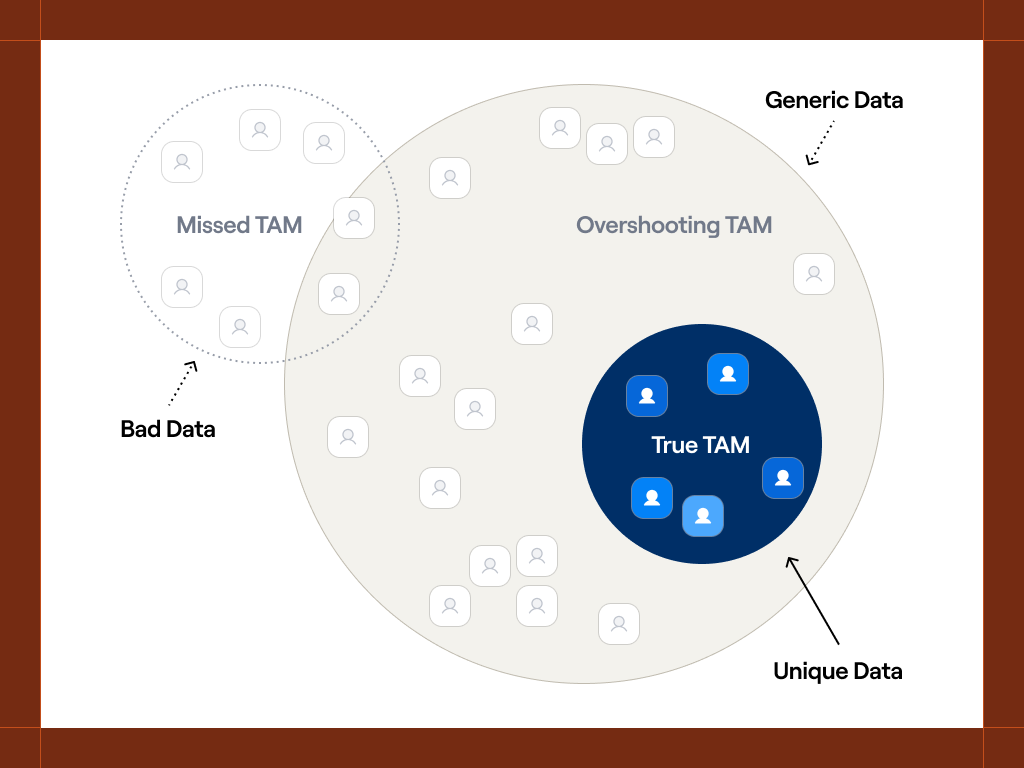
Part 2: Experiment with high-alpha plays
It's what you do with the data that makes winning teams. After you find unique data with AI, you should deploy it in unique plays. Below are some examples from our customer base (see Claybooks for more):
- Verkada auto-generates thousands of personalized landing pages for good-fit prospects, using individual company logos and information.
- Rippling uses Google Maps to find prospects' possible corporate addresses & calculates commuting distances to identify which location is most likely the active office to reach for direct mail
- An AI marketplace monitors rising products on Amazon and Walmart in real-time and automatically contacts sellers when their products gain traction to offer pricing and ad management. For international sellers, they search on foreign marketplaces and translate content with AI.
We see new examples all the time at our AI GTM hackathons, where we help customers identify unique data points and launch new plays in hours. Last week, for example, Vanta automated a system to generate business review slideshows with customer health metrics and product usage data.
Continuous experimentation is the key to maintaining GTM alpha. Your plays must change as customer buying patterns evolve or you enter new markets. Your GTM should be like a science, where you hypothesize, test, learn, and iterate.
GTM teams could traditionally only test a few plays per quarter because finding quality data, combining it from disparate sources, and deploying it into workflows was painfully slow and required many disconnected tools. Modern technology has changed this equation.
Today’s winning teams use GTM development environments: platforms where you can both source data and run plays in one place. With a few clicks, you can create precise customer segments, adjust qualification criteria, and deploy campaigns with varied messaging.
GTM alpha comes from launching and testing unique plays faster than your competitors. By the time competitors catch on, you should have moved on to your next set of experiments.

Part 3: Build a GTM engineering organization
Organizations that find GTM alpha look different than traditional sales teams.
Traditional GTM organizations operate like assembly lines: SDRs prospect, AEs close deals, and RevOps manage systems. But assembly lines are designed for standardization, not learning.
You can’t quickly find and scale winning tactics in silos where SDRs prospect & message leads, AEs close deals, and RevOps manage systems. Consider an SDR who realizes that companies that raised a Series B and are hiring salespeople respond well to compliance risk messaging. She crafts a successful email campaign, but her insights don’t spread to other SDRs. Her RevOps team, focused only on CRM maintenance, doesn’t help scale her approach.
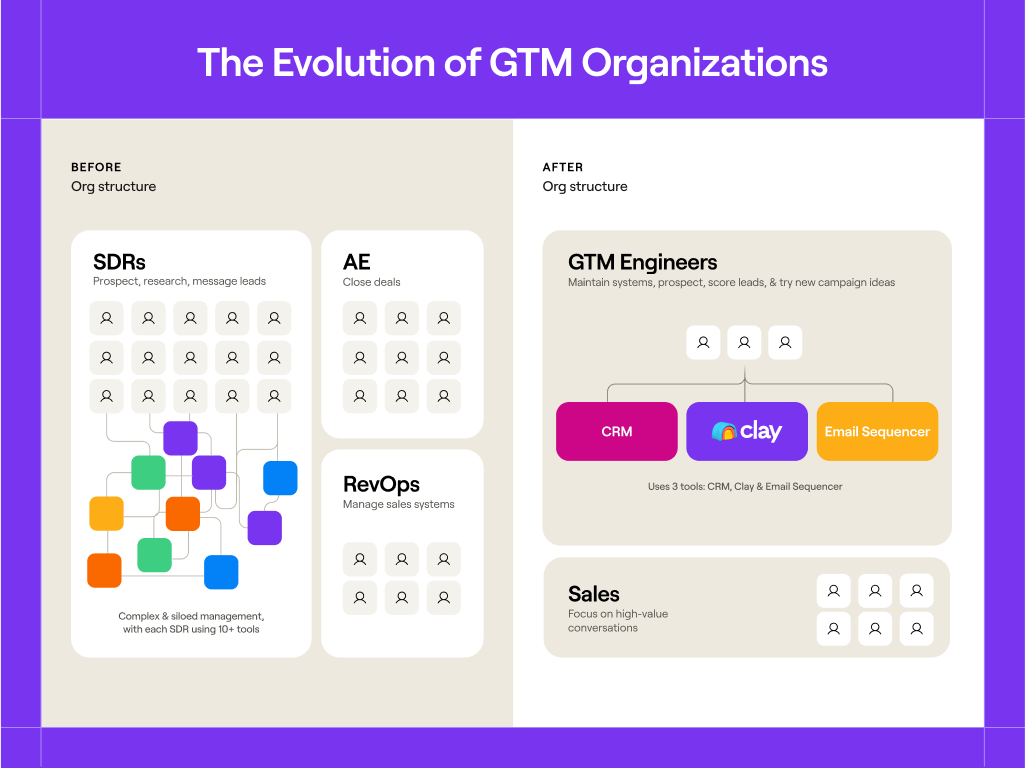
Forward-thinking companies adopt a GTM engineering approach. The essence of GTM engineering is centralizing technical expertise and sales knowledge to scale revenue. Different organizations implement this in different teams.
For example, in Anthropic’s Sales Ops team, Adam Wall uses Clay to automate lead enrichment, scoring and routing. Salespeople then can focus just on high-value conversations with qualified prospects. This is more than just a time-savings effort: the GTM Engineering and the AI workflows that the RevOps team implements with Clay aligns with their mission to send teams more powerful data and make them more efficient.
At Clay, our GTM Engineering team aims to build an always-on engine that centralizes, cleans, and processes data from various sources, including Gong calls, website visits, and product data—and turning it into action. The goal is for salespeople to do no manual data entry, summarization, or cleaning.

Regardless of how you get there, the goal of GTM engineering is to build teams that can find, test, and scale new approaches faster than competitors.
The continuous pursuit of GTM alpha
This is the reality of modern growth: there is no permanent competitive advantage, only the continuous pursuit of temporary advantages. The market evolves, competitors copy successful tactics, and buyers become numb to approaches they've seen before. The best teams aren't attached to their current methods—they're energized to discover what works next. A team that knows how to learn is a team that wins.
Gimmicks fade, but what always works is addressing your prospects' challenges better than anyone else. Differentiated GTM means better data, better playbooks, and constant experimentation. Pair that with a solid product, and you've built a winning company.
*
Many thanks to Rachel Hepworth, Stevie Case, Gaurav Vohra, Arielle Jackson, Dakota McKenzie, Kim Graves, Renu Gupta, Petra Hajal, Adam Wall, Jen Iguarta, Emily Miller, Kris Rudegraap, Alexander Demoulin, Andrew Thomas, and Dannie Herzberg for feedback on this piece!
Winning teams see things others don't and do things others can't.
In finance, "alpha" is an investment’s outperformance over a market benchmark. It's what separates market-beating investors from the rest of the pack. Every GTM team is constantly seeking alpha—even if you don’t call it that yet. Each time you refine your targeting or messaging to beat your competitors, you're chasing alpha.
And just like investors, winning GTM teams use data others don't have—in plays others can’t run—to find an edge.
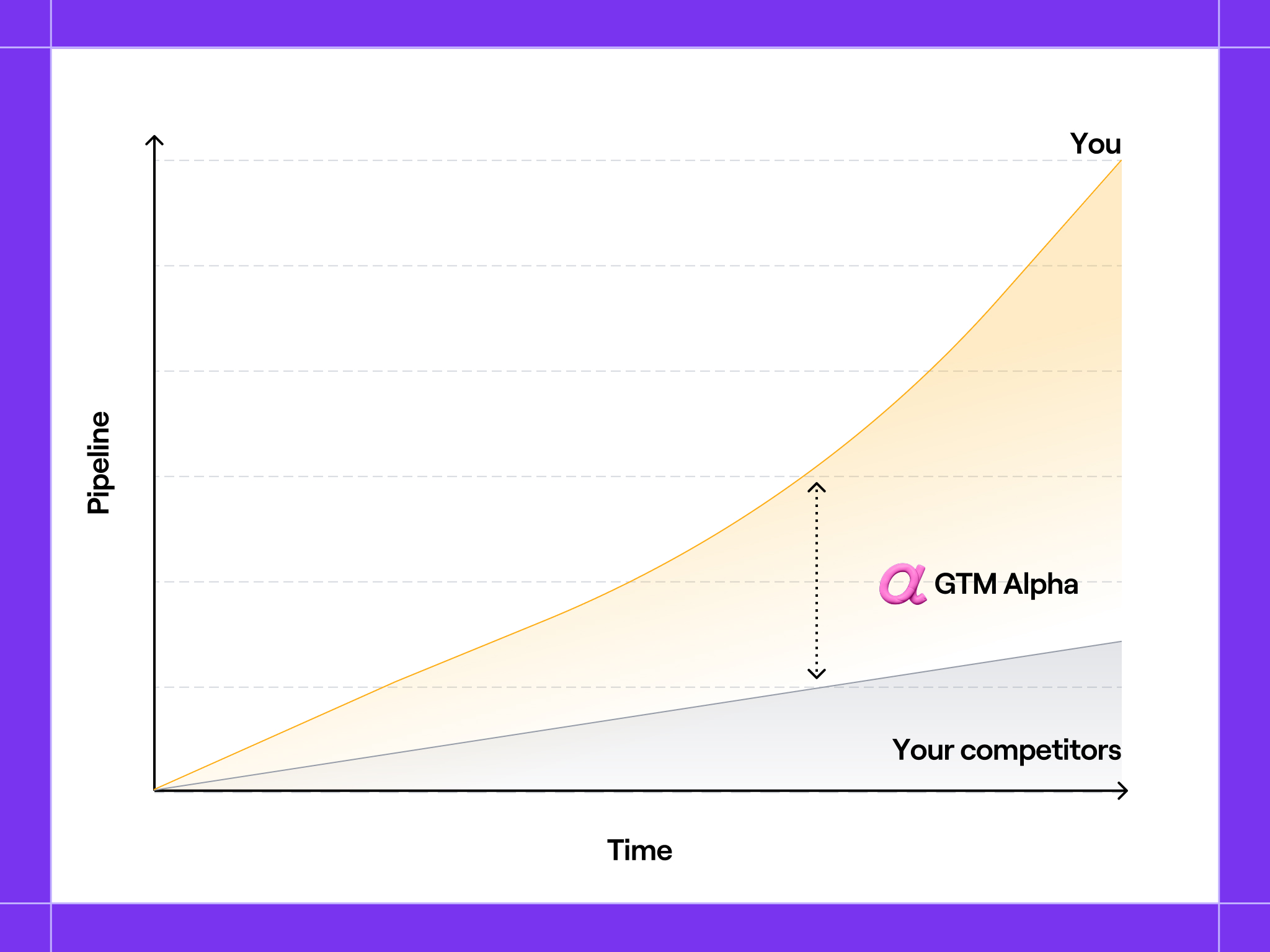
GTM alpha starts with better data than your competitors. Accurate, comprehensive, and timely data is table stakes, though most companies don’t even get this far. (If you can’t even trust the email addresses or firmographics in your CRM, fix that first.)
Unique data, however, is your competitive edge. While competitors blast messages to "all restaurants in Europe," you should be pinpointing Berlin cafes with $30-50 entrees that just joined Doordash. Instead of targeting generic SaaS companies, you should be finding those with usage-based pricing, free trials, and newly posted customer success jobs. Unique data gives you a precision edge.
Study your best customers to know what unique data points to look for. AI agents can find any data point at scale by reading websites or documents to categorize or summarize information. They can, for example, infer whether a company has specific certifications or exceeds a certain growth rate.
Armed with unique data, winning teams can run unique plays. Plays based on surface-level personalizations—like mentioning someone's college or referencing website visits—never work for long. Exceptional performers do it differently. A global design company, for example, has thought about using AI to find brand inconsistencies between prospects' websites and social media accounts and suggest solutions—an approach that performs twice as well as generic outreach.
Continuous experimentation is the key to maintaining alpha. No play lasts forever: the market and your customers are constantly changing. Top teams stay ahead by experimenting, learning, and launching better plays faster. By the time competitors reverse-engineer a strategy that worked for you last year, you should be on to your next one.

Part 1: Find your unique data advantage
When you and your competitors only target based on company size, industry, and job titles, you're all going to be selling to the same companies at the same time. Unique AI data—tailored to your company’s product and value proposition—will help you more precisely narrow in on accounts that have a burning need for your product. These data points are difficult to find, which means BDRs often spend their time manually researching them. But AI agents can systematically collect these qualitative & unstructured data points. They're the hidden signals that your competitors can't see because they don't know to look for them.
To decide what unique data points you need, the first step is to deeply understand your customers. Interview your sales team about what they manually research before calls and why those signals matter. Listen to where prospects are having conversations and what language they use. Map their digital footprint: what they read, where they gather, what tools they mention, and what problems they discuss. If you had 100 interns, what would you have them do? If you could reach out to a company at any specific time, when would it be? Most companies we’ve met, including market leaders, do not put nearly enough scrutiny into this.
The next step is turning these indicators of customer fit into unique data points you can get with AI. Ideally, this combines public data, 1st party data, as well as custom signals. For example, when a prospect told us they land the most deals right before businesses enter promotion cycles, we suggested they use AI to find employee promotion date clusters and map these to different firms.
Here are examples of how companies are using Clay’s AI agent to discover unique data points their competitors can't see:
- Certemy counts OSHA violations to find companies with compliance problems
- Supermetrics distinguishes between brands and agencies to route leads to the right sales teams
- Rutter uses AI to identify high-value executives who need financial products the moment relevant conference attendee lists become public
- Intercom determines the breadth of a company’s support documentation
- Cake.ai identifies AI engineering teams that have 2-5 developers (their sweet spot)
Other customers try novel ideas like scanning satellite images to analyze building locations for indicators of occupancy, tracking where prospects are expanding office locations, etc.
Unique data is most powerful when paired with precise timing. Markets change daily, and signals often have short windows of relevance. Companies that quickly capture and act on time-sensitive data consistently outperform those relying on monthly or quarterly refreshes.
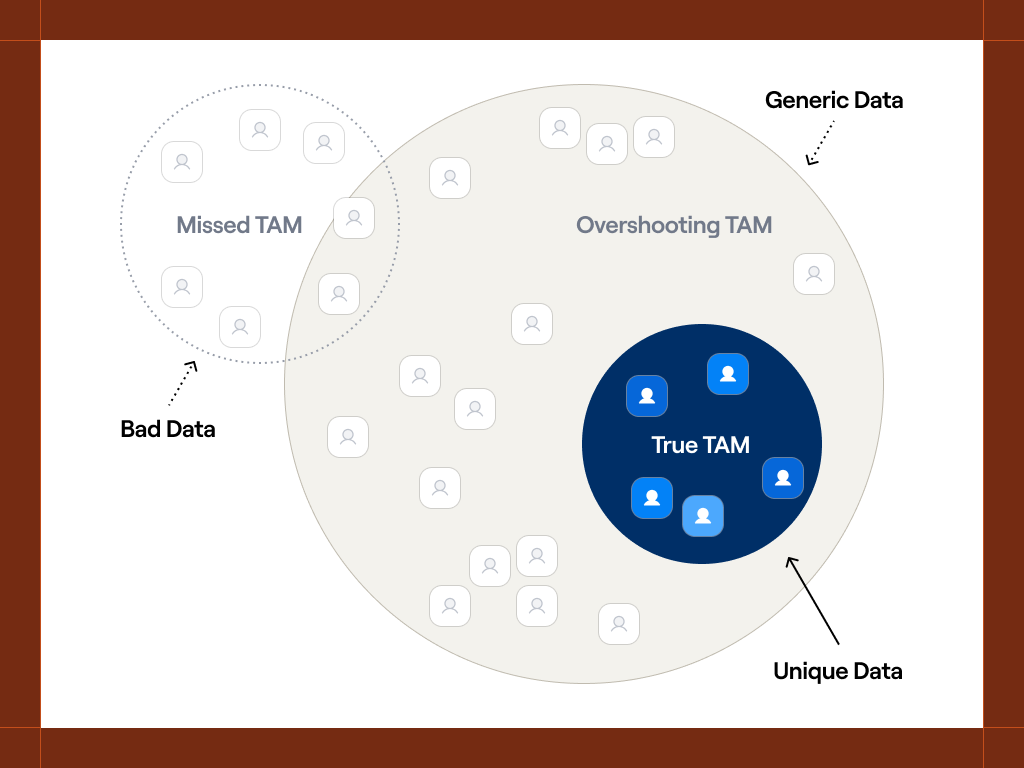
Part 2: Experiment with high-alpha plays
It's what you do with the data that makes winning teams. After you find unique data with AI, you should deploy it in unique plays. Below are some examples from our customer base (see Claybooks for more):
- Verkada auto-generates thousands of personalized landing pages for good-fit prospects, using individual company logos and information.
- Rippling uses Google Maps to find prospects' possible corporate addresses & calculates commuting distances to identify which location is most likely the active office to reach for direct mail
- An AI marketplace monitors rising products on Amazon and Walmart in real-time and automatically contacts sellers when their products gain traction to offer pricing and ad management. For international sellers, they search on foreign marketplaces and translate content with AI.
We see new examples all the time at our AI GTM hackathons, where we help customers identify unique data points and launch new plays in hours. Last week, for example, Vanta automated a system to generate business review slideshows with customer health metrics and product usage data.
Continuous experimentation is the key to maintaining GTM alpha. Your plays must change as customer buying patterns evolve or you enter new markets. Your GTM should be like a science, where you hypothesize, test, learn, and iterate.
GTM teams could traditionally only test a few plays per quarter because finding quality data, combining it from disparate sources, and deploying it into workflows was painfully slow and required many disconnected tools. Modern technology has changed this equation.
Today’s winning teams use GTM development environments: platforms where you can both source data and run plays in one place. With a few clicks, you can create precise customer segments, adjust qualification criteria, and deploy campaigns with varied messaging.
GTM alpha comes from launching and testing unique plays faster than your competitors. By the time competitors catch on, you should have moved on to your next set of experiments.

Part 3: Build a GTM engineering organization
Organizations that find GTM alpha look different than traditional sales teams.
Traditional GTM organizations operate like assembly lines: SDRs prospect, AEs close deals, and RevOps manage systems. But assembly lines are designed for standardization, not learning.
You can’t quickly find and scale winning tactics in silos where SDRs prospect & message leads, AEs close deals, and RevOps manage systems. Consider an SDR who realizes that companies that raised a Series B and are hiring salespeople respond well to compliance risk messaging. She crafts a successful email campaign, but her insights don’t spread to other SDRs. Her RevOps team, focused only on CRM maintenance, doesn’t help scale her approach.
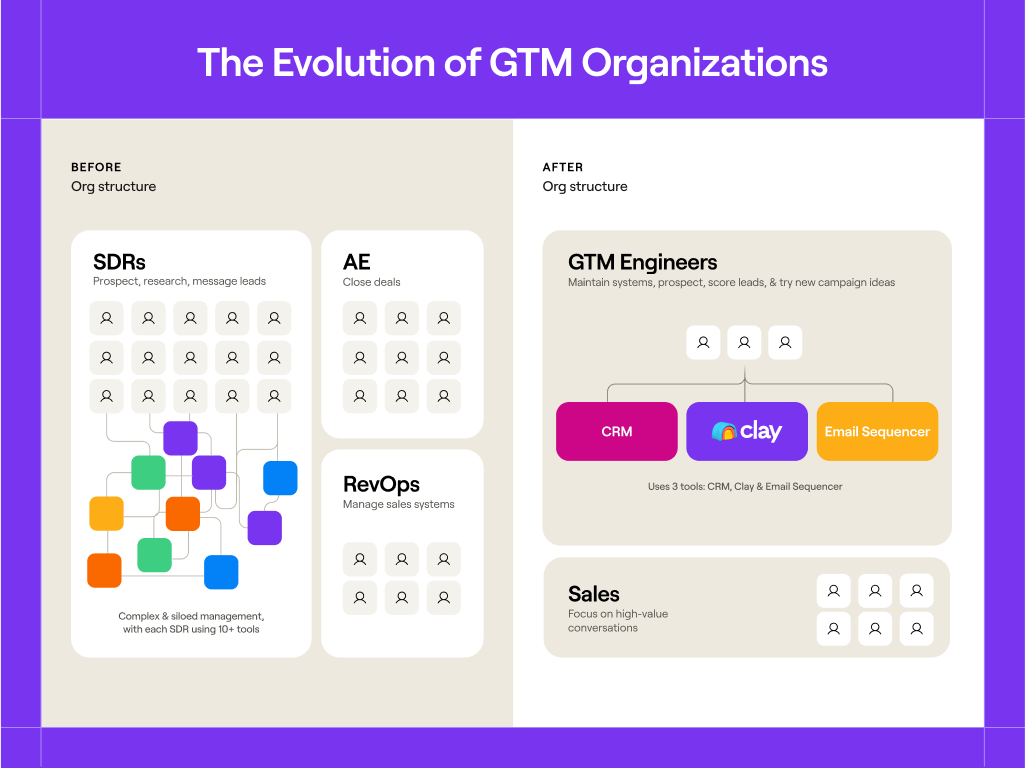
Forward-thinking companies adopt a GTM engineering approach. The essence of GTM engineering is centralizing technical expertise and sales knowledge to scale revenue. Different organizations implement this in different teams.
For example, in Anthropic’s Sales Ops team, Adam Wall uses Clay to automate lead enrichment, scoring and routing. Salespeople then can focus just on high-value conversations with qualified prospects. This is more than just a time-savings effort: the GTM Engineering and the AI workflows that the RevOps team implements with Clay aligns with their mission to send teams more powerful data and make them more efficient.
At Clay, our GTM Engineering team aims to build an always-on engine that centralizes, cleans, and processes data from various sources, including Gong calls, website visits, and product data—and turning it into action. The goal is for salespeople to do no manual data entry, summarization, or cleaning.

Regardless of how you get there, the goal of GTM engineering is to build teams that can find, test, and scale new approaches faster than competitors.
The continuous pursuit of GTM alpha
This is the reality of modern growth: there is no permanent competitive advantage, only the continuous pursuit of temporary advantages. The market evolves, competitors copy successful tactics, and buyers become numb to approaches they've seen before. The best teams aren't attached to their current methods—they're energized to discover what works next. A team that knows how to learn is a team that wins.
Gimmicks fade, but what always works is addressing your prospects' challenges better than anyone else. Differentiated GTM means better data, better playbooks, and constant experimentation. Pair that with a solid product, and you've built a winning company.
*
Many thanks to Rachel Hepworth, Stevie Case, Gaurav Vohra, Arielle Jackson, Dakota McKenzie, Kim Graves, Renu Gupta, Petra Hajal, Adam Wall, Jen Iguarta, Emily Miller, Kris Rudegraap, Alexander Demoulin, Andrew Thomas, and Dannie Herzberg for feedback on this piece!